LG Aimers 학습 내용을 정리한 글입니다.
Last lecture : how good is a specific policy ?
Thid lecture : how can we learn a goof policy ?
- Explore : take actions haven't tried much in a state
- Exploit : take actions estimate will yield high discounted expected reward
1. ε-greedy Policy → Explore과 Exploit의 balance문제를 해결하는 가장 간단한 알고리즘
: ε을 probability로 random하게 action을 고르는 것 (모든 행동들이 0이 아닌 확률로 수행된다.)
Monte-Carlo Policy Iteration
- Policy evaluation : Monte-Carlo policy evaluation
- Policy improvement : ε-greedy Policy improvement
→ takes too much time/samples
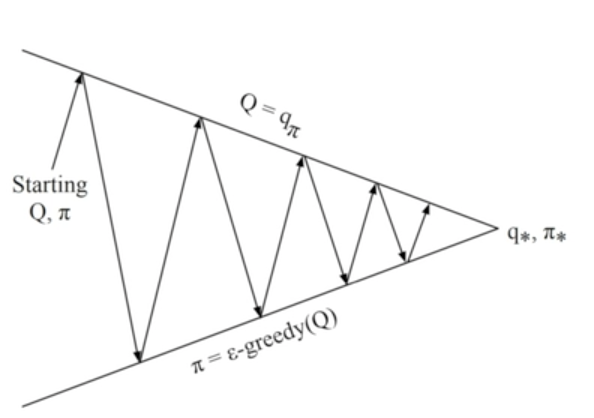
Monte-Carlo Control : Every episode
- Policy evaluation : Monte-Carlo policy evaluation
- Policy improvement : ε-greedy Policy improvement
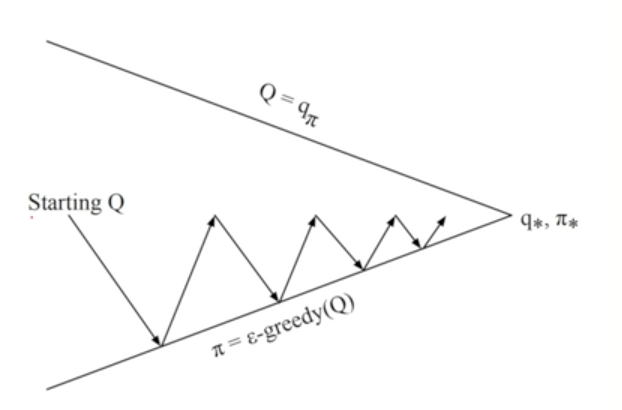
Greedy in the Limit of Infinite Exploration(GLIE)
Model-free Policy Iteration with TD Methods : ε-greedy Policy가 GLIE 컨디션을 만족하도록 Scheduling 해 주는 알고리즘
2. SARSA 알고리즘 : Policy Iteration
→ Optimal Q function찾기
→ on-policy learning algorithm : estimates the value of the behavior policy
Stochastic approximation : learning rate를 두고 기존에 Q를 잔차 만큼 계속해서 update해 나가는 알고리즘
3. Q-Learning 알고리즘 : Learning the Optimal State-Action Value : Value Iteration
→ SARSA와 비슷하지만, policy improvement step을 추가적으로 두고 있지 않다.
→ off-policy RL algorithm : Target policy ↔ Behavior policy
→ No importance sampling
→ maximization bias도 나타날 수 있음 →Double Q-Learning으로 해결
- On-policy learning
- Direct experience
- 실제로 행동을 하면서 학습 - Off-policy learning
- 누군가가 따로 실제 상황에서 행동을 하고, 그 행동 하는 것을 관측하면서 다르게 학습

- Importance Sampling 기법
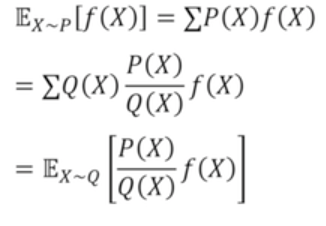
비교
'Add, > LG Aimers' 카테고리의 다른 글
[LG Aimers] 강화학습5 - Deep Q Learning (0) | 2023.07.29 |
---|---|
[LG Aimers] 강화학습4 - Function Approximation (0) | 2023.07.28 |
[LG Aimers] 강화학습2 - Model-Free Policy Evaluation (0) | 2023.07.27 |
[LG Aimers] 강화학습1 - MDP and Planning (0) | 2023.07.26 |
[LG Aimers] 지도학습(분류/회귀)5 - Ensemble/Evaluation (0) | 2023.07.26 |
댓글