[LG Aimers]Deep Neural Networks 딥러닝5 - Transformer/Self-supervised Learning/Large-Scale Pre-Trained mode
LG Aimers 학습 내용을 정리한 글입니다
1. Transformer 모델의 동작 원리
: attention module can work as both a sequence encoder, and a decoder in seq1seq with attention.
- Long-term Dependency lssue of RNN models
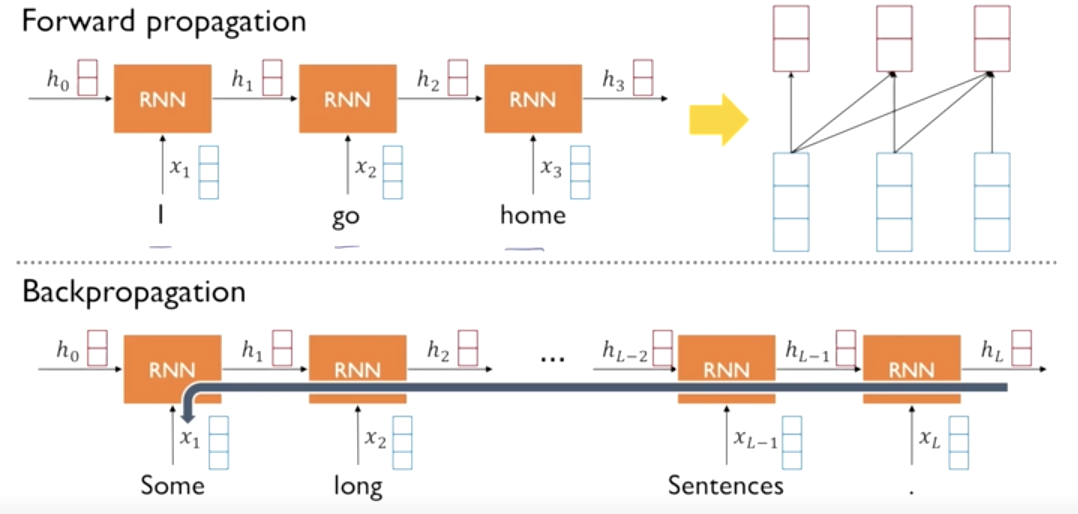
→ Long-term dependency 문제를 해결한다.
Scaled Dot-product Attention
Multi-head Attention
Masked self-attention
2. 자가지도학습 및 언어 모델을 통한 대규모 사전 학습 모델
self-supervised learning : given unlabeld data, hide part of the data and train the model so that it can predict such a hidden part of data, given the remaining data.
Transfer learning from self-supervised pre-trained model target task.
: pre-trained models using a particular self-supervised learning can be fine-tuned to improve the accuracy of a given
- BERT : pre-trainig of deep Bidirectional Tranformers for language understanding
→ masked language modeling(MLM) and next-sentence prediction(NSP) task로 학습
- Masked Language model(MLM) : mask some percentage of the input tokens at random, and then predict dhose masked tokens.
- Next Sentence prediction(NSP) : predict whether sentence B is an actual sentence that follows sentence A, or a random.
- GPT-1/2/3 : Generative pre-trained transformer(decoder)
→ language model 로 많은 양질의 데이터로 학습
→ GPT 2: zero-shot summarization ( predict the answer given only a natural language description of the task)
→ GPT 3: few-shot learning (see a few examples of the task)
정확한 이해도 어렵지만 이해한 것을 정리하기 어려움이 컸다,, 추가로 공부해서 더 정리를 해야겠다.